ABOVE: modified from © istock.com, Yana Lysenko, mathisworks; designed by erin lemieux
In 2015, Jennifer Byrne, a cancer researcher at the University of Sydney, noticed something strange while browsing papers related to her past research. A handful of papers recently published by separate research groups had all linked the expression of a gene that she had cloned in the 1990s with different types of cancer. Byrne, who had studied cancer-associated genes for more than two decades, recalled, “That struck me as strange because for many years no one had been interested in this gene.” In fact, in Byrne and her colleagues’ investigation of the gene, they realized early on that there was limited evidence for this gene as an important driver of cancer development. “If we, as the people who cloned the gene, weren't interested in the gene in cancer, well, why would anyone else be?” she wondered.
When she looked into the details of the papers, including the methods and materials sections, she noticed several mistakes in the nucleotide sequences.1 “[The nucleotide sequences] weren't peripheral to the research; they were absolutely core to the research, so if they were wrong, everything was wrong,” said Byrne.
Byrne was shocked; she wanted to know what was going on. “That's what we've been trained to do, so that's what I did. As I dug, I realized that there were a lot more of these papers,” she said.
As I dug, I realized that there were a lot more of these papers.
—Jennifer Byrne, University of Sydney
For Byrne and the community of scientists and sleuths who were already struggling to manage a growing pollution problem in the scientific literature a few years ago, the problem is only getting worse. Many fear that the recent emergence of artificial intelligence (AI) tools will make it easier to generate, and harder to detect, fraudulent papers. New tools are aiding efforts to flag problematic papers, such as those riddled with image issues, nonsensical text, and unverifiable reagents, but as deception techniques become more sophisticated, the countermeasures must evolve to keep pace. Scientists are turning to AI to fight AI, but current detection tools are far from being the panacea that is needed.
With problematic papers on the rise, will scientists be able to tell whether they are standing on the shoulders of giants or propped up on feet of clay?2
Detecting Fingerprints of Plagiarism and AI-Generated Text
Over the last decade, Byrne has gradually shifted her research focus from cancer genetics to the science integrity issues that she saw plaguing her field. However, it’s difficult to prove that a paper is fabricated; it’s expensive and time consuming to replicate every experiment covered in a paper. “That's why we're looking at shortcuts,” said Byrne.
Following her discovery of suspiciously similar cancer papers, Byrne teamed up with computer scientist Cyril Labbé at Grenoble Alps University to develop tools to automate the detective work that she was doing by hand. Alongside their program that verifies the identities of nucleotide sequences, they also developed a tool that detects unverifiable human cell lines.3,4 These tools were integrated into a larger program called the Problematic Paper Screener, which is spearheaded by Guillaume Cabanac, an information scientist at the University of Toulouse.
Cabanac started working on the Problematic Paper Screener with Labbé back in 2020 to detect grammatical patterns in text produced by popular random paper generators like SCIgen and Mathgen, which generate professional-looking computer science or mathematics papers, respectively. However, upon closer examination, the papers are nonsensical and follow a templated writing style. “We would use that as fingerprints, like in a crime scene,” said Cabanac. Since then, the program has expanded to include several detection tools, including a tortured-phrases detector, which flags papers that contain weird strings of text that the paraphrasing tool SpinBot uses in lieu of well-established scientific terms, such as “bosom disease” for breast cancer and “counterfeit consciousness” for artificial intelligence.5 However, by the time researchers developed new methods to detect these indicators of fraud, research misconduct had already begun to evolve.
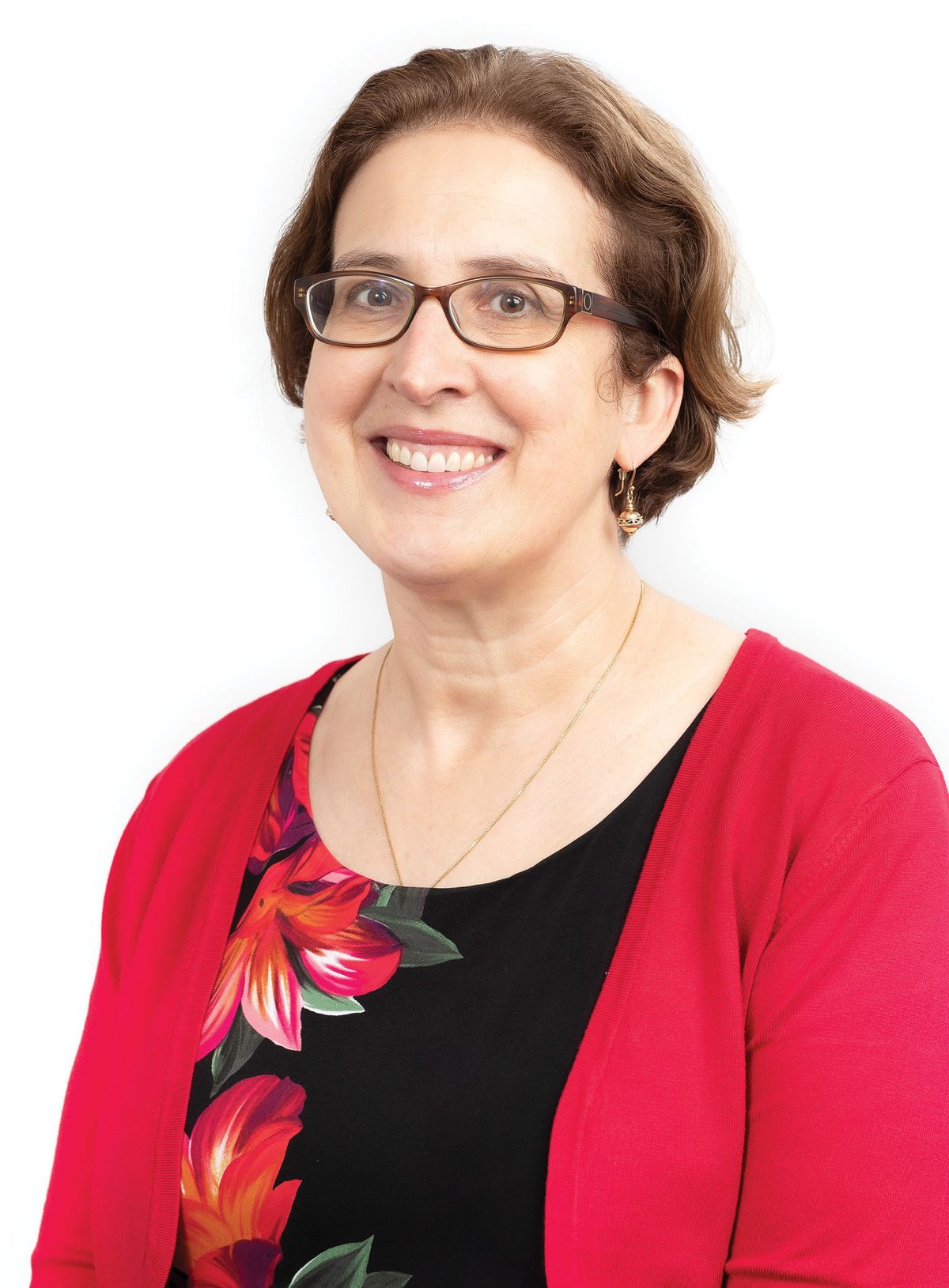
Just a couple of years later, ChatGPT, OpenAI’s large language model (LLM), was released. Now, anyone can feed the virtual writing assistant successive prompts to generate and refine text that looks human-like and lacks the classic plagiarism fingerprints that researchers have been using to detect problematic papers. “They are much more clever,” said Cabanac. “They produce really good text.”
As LLMs and AI content generators produce increasingly sophisticated and convincing text, the tools that scientists have been relying on to detect scientific fraud may soon become obsolete. “We have found that now increasingly the papers are getting much more complex, or at least the ones that we study are getting more complex,” said Byrne.
Although there is still an ongoing debate on whether AI-generated text is plagiarism, this is not the only concern scientists have when it comes to handing off publication preparation to an LLM. Currently, LLMs suffer from hallucinations, generating text that is grammatically correct but otherwise nonsensical, misleading, or inaccurate.6 Therefore, human oversight is still necessary to weed out fake findings and citations and prevent the spread of falsehoods. Many fear that there is already wide-scale abuse of LLMs by paper mills to produce fraudulent papers riddled with unreliable science, but detecting AI-generated content, which is trained on human text, is tricky.
Because of copyright restrictions, the training data sets for LLMs are largely restricted to old texts from the early twentieth century. As a result, some researchers have used the frequency of certain words that were popular then but have since fallen out of common parlance as evidence of generative AI. However, according to Cabanac, this is not definite evidence; he prefers looking for obvious fingerprints. In the summer of 2023, only half a year after ChatGPT reached the masses, he found them popping up in the literature. “I found some evidence—some smoking guns—related to the use of ChatGPT in scientific publications,” said Cabanac.
For example, when prompted to generate text on the future directions of the research, the chatbot might begin the response with ‘As an AI language model, I cannot predict the future,’ and these statements were ending up in published papers. “I found that this is really appalling because it means that peer review, in this case, didn't catch this evident problem,” said Cabanac.
Detecting Research Misconduct in the Age of Artificial IntelligenceIn 2023, academic journals retracted nearly 14,000 papers, up from around 2,000 a decade before. Plagiarism and concerns about data authenticity accounted for most of the cases, with many papers showing clear "fingerprints" of research misconduct. ![]() For years, the literature has been inundated with papers featuring nonsensical text, unusual phrases, unverifiable reagents, and manipulated images. Now, there is growing evidence of content generated by large language models and artificial intelligence (AI)-based systems.1-3 ![]() To counteract the rising number of fraudulent papers and depollute the literature, scientists are developing tools that detect these “fingerprints,” with emerging detection tools pitting AI against AI. ![]() modified from © istock.com, mathisworks; designed by erin lemieux However, as fraudsters continually develop new methods to outsmart existing safeguards, detection tools need to evolve faster to keep up with deception. |
Sharpening the Lens: AI Image Manipulation Comes into Focus
Images, an essential element of review and original research papers, are not immune to the wiles of tricksters looking to deceive. Those who frequent social media platforms may remember a much-discussed graphic depicting a rat with massive genitalia that made the rounds. It contained nonsensical labels, such as “sterrn cells,” “iollotte sserotgomar,” and “dissilced,” and appeared alongside other questionable figures in a paper published in the journal Frontiers in Cell and Developmental Biology. (The journal has since retracted the paper, noting that it did not meet the journals “standards of editorial and scientific rigor.”)
The botched image was a rude awakening for scientists that generative AI had entered the scientific literature. Many warn that this is just the tip of the iceberg. It is already becoming harder to distinguish, by human eye, a real image from a fake, AI-generated one.
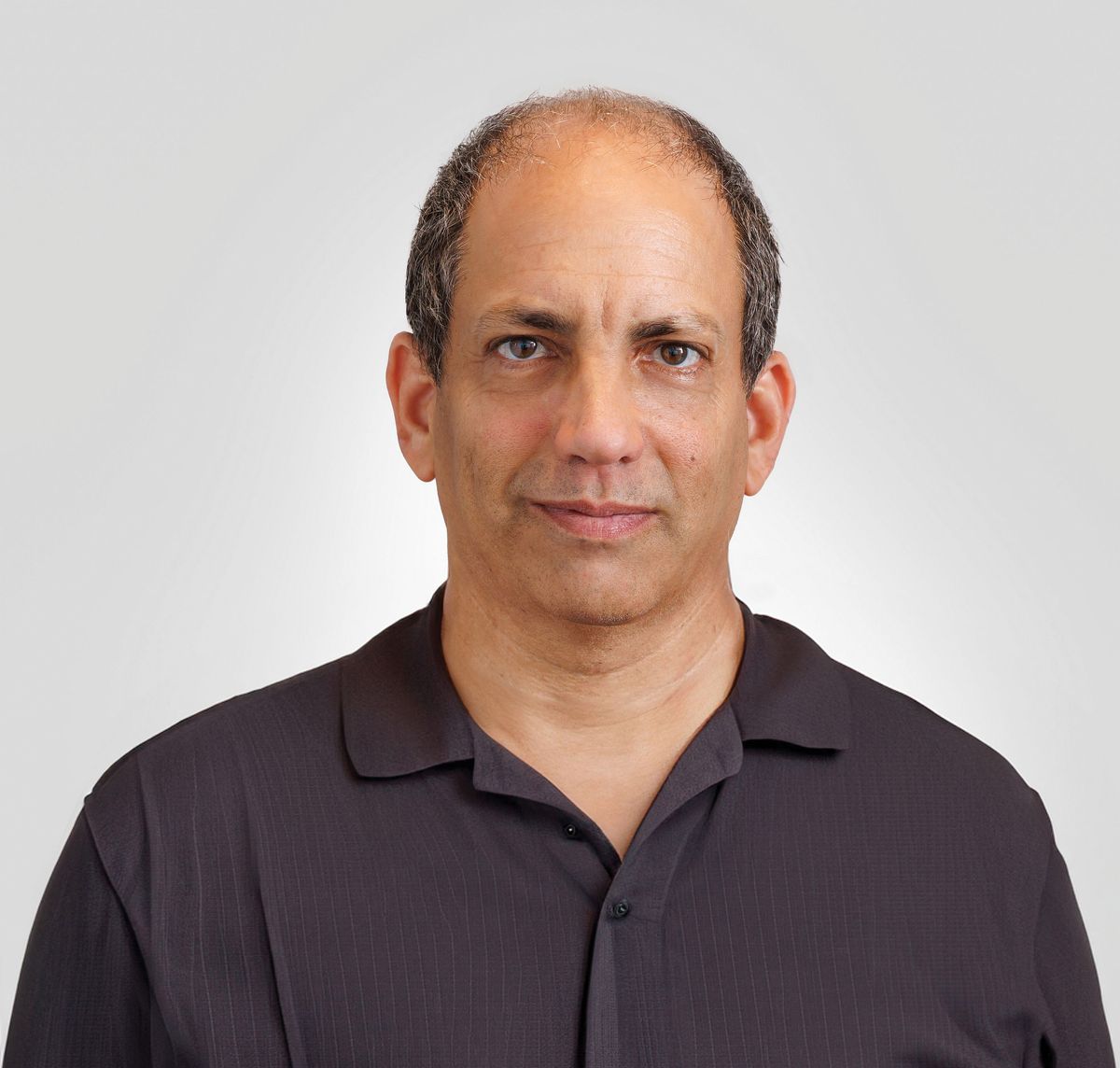
“There were always people that try to deceive and use technology,” said Dror Kolodkin-Gal, a scientist-turned-entrepreneur and founder of Proofig AI (previously called Proofig), a company that provides image analysis tools. Kolodkin-Gal noted that while people have used software to manipulate figures previously, “It's really scary at the same time that the AI can generate something that looks so real.”
Back in the early 2010s, Kolodkin-Gal was working at Harvard Medical School as a postdoctoral researcher when he realized that there were no tools available for authenticating images like there were for checking plagiarism in text. “We are doing so much work [as researchers], days and nights, many experiments, and we do not have quality assurance in terms of images,” said Kolodkin-Gal. “That quite surprised me.” Kolodkin-Gal also knew firsthand how easy it was to mix up microscopy images when sifting through hundreds of similar images. Luckily, he said, he caught his mistake prepublication. “I got it in the back of my head, remembering this experience—it could happen by innocent mistake.” But not everyone he knew was as lucky, and many were left to deal with post-publication headaches. “Post publication—I call it postmortem,” said Kolodkin-Gal. “It's too late.”
Kolodkin-Gal decided to fill this technological gap by developing a tool that researchers and journals could use to detect potential image issues, including reuse, duplication, and manipulation. In 2020, Proofig AI, an AI-powered image analysis tool with the tagline “Publish with peace of mind,” was born.
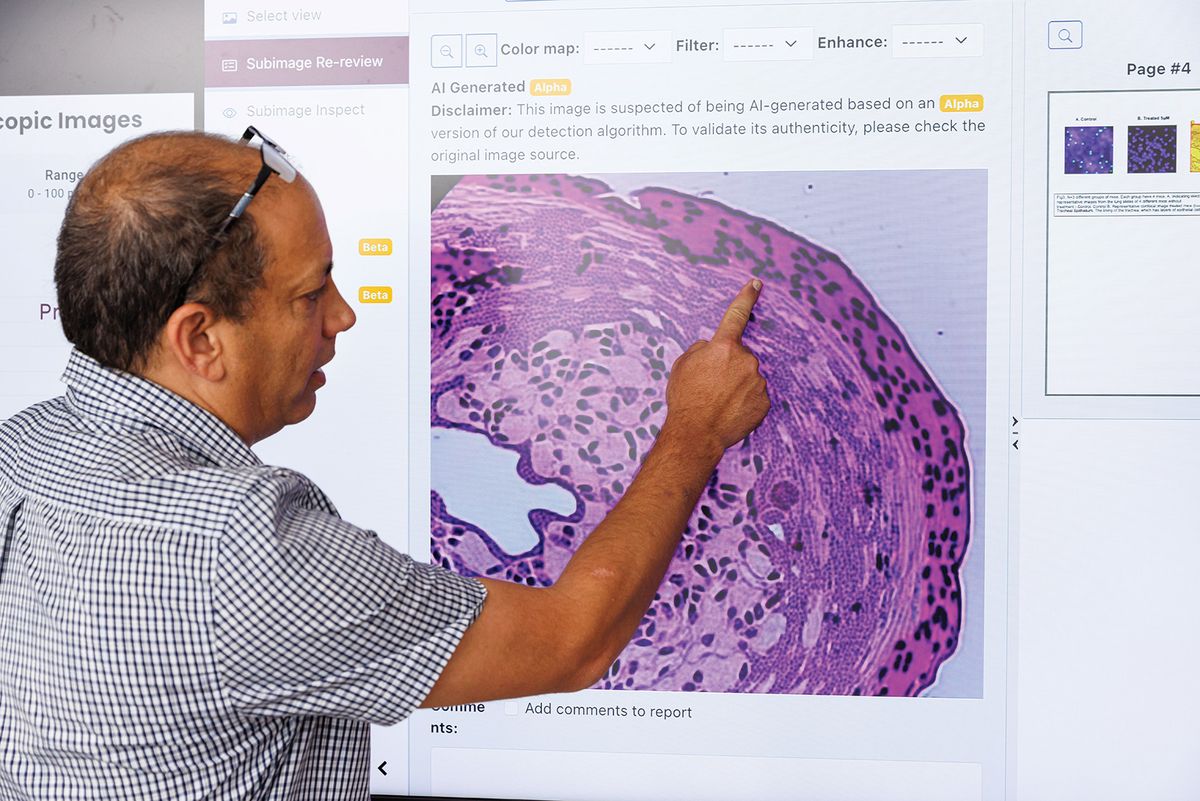
Now, with a click of a button, forensics work, such as image contrasting to detect deleted bands in a western blot, that takes science sleuths hours to perform manually can be performed nearly instantly and at scale. Proofig AI also performs tasks that are much harder for humans: The AI tool can compare images in a document to the millions of online images that are open source for commercial use. Currently, images tucked behind paywalls are beyond the visibility of the tool, but Kolodkin-Gal said that when it comes to paper mills looking to fabricate images for a publication, they don't need this old data. “They will produce it by using AI,” he added. “It's more important to focus on the AI generated [content].”
Science sleuths and scientific journals have started to integrate AI-based image analysis tools like Proofig AI, ImageTwin, and FigCheck for detecting image integrity issues. The American Association for Cancer Research started using Proofig AI in 2021, and earlier this year the editor-in-chief of Science journals announced that they would begin using the tool across all six of their journals.
While comically bad diagrams of the male rat anatomy are on one end of the spectrum, worryingly realistic AI-generated microscopy images occupy the other end. Recently, Kolodkin-Gal and his team released a new version of their tool that they say can detect AI-generated images. “It's like an evolution of virus and antivirus. It's an AI identifying AI—the good AI, identifying the bad AI,” said Kolodkin-Gal.
False Positives are a Big Negative for AI Detection Tools
Vinu Sankar Sadasivan, a fourth-year computer science graduate student, works with computer scientist Soheil Feizi at the University of Maryland, College Park on security and privacy in generative AI models. To probe weaknesses in LLMs like ChatGPT, he plans adversarial attacks that are meant to trick or deceive the chatbots into revealing security risks that they are currently designed to keep hidden, such as personal information that other users have used for prompts. “It's important for people to attack systems, to know their vulnerabilities,” said Sadasivan. Even though the chance of real adversaries attacking such systems is very small, he added, “You should be aware of that, because people could misuse such technologies which are very powerful.”
It's like an evolution of virus and antivirus. It's an AI identifying AI—the good AI, identifying the bad AI.
—Dror Kolodkin-Gal, Proofig AI
In response to the potential harm of text and images generated by LLMs, new AI-powered AI-recognition tools are cropping up. To help prevent misuse of their LLM services, companies like Google, Meta, and OpenAI are exploring different security systems that will facilitate the identification of AI-generated content. Although security approaches differ depending on the modalities, Sadasivan said that watermarking, trained neural networks (machine learning models that, inspired by the structure and function of the human brain, use interconnected nodes for information processing), and retrieval-based techniques are some of the methods commonly used for detecting both AI-generated text and images. Although many of these approaches are effective, Sadasivan said that they are not without pitfalls. Some people working on these issues worry that these measures could limit the quality of the content produced and that tasks with a limited number of possible output options will exhibit high overlap between what a human would write and what the AI generates. “It's actually an inherent challenge with the text modality itself, which is why it is a more difficult modality than images, because it's discrete domain and it's very hard to add some signal to it to make it still look meaningful, and make it easier to be detected,” said Sadasivan.
Another limitation of relying on these front-end methods that are employed by the AI generator is that these security methods must be broadly adopted for success. “If you have watermarking, you have to make sure that all the best generators that exist should be watermarked,” said Sadasivan. “Otherwise, people would just use the generator without a watermark in it.” Also, in response to these countermeasures, he said that companies are already offering services that promise to remove watermarks, using AI to hide AI from AI.
It’s important to be able to identify AI-generated content to prevent misuse, such as creating fake scientific findings, but Sadasivan and others working in this area have shown how detectors are unreliable. He and his colleagues planned adversarial attacks on detectors that rely on watermarking schemes and found that they are vulnerable to attacks meant to mislead detectors, causing them to falsely label human-written text as AI-generated.7 Detection tools that use neural networks trained on human and AI-generated content to reliably classify materials present an alternative, post hoc method, but they suffer from high false positive rates and are subject to false negatives if they are presented with new AI-generated material that the LLM has not been trained on. “From our paper, what we see is [that] these kinds of detectors are one of the worst to be used, and they can be easily broken,” said Sadasivan. In what many viewed as a major setback for the field, in July 2023, OpenAI retracted its AI classifier due to a low rate of accuracy.
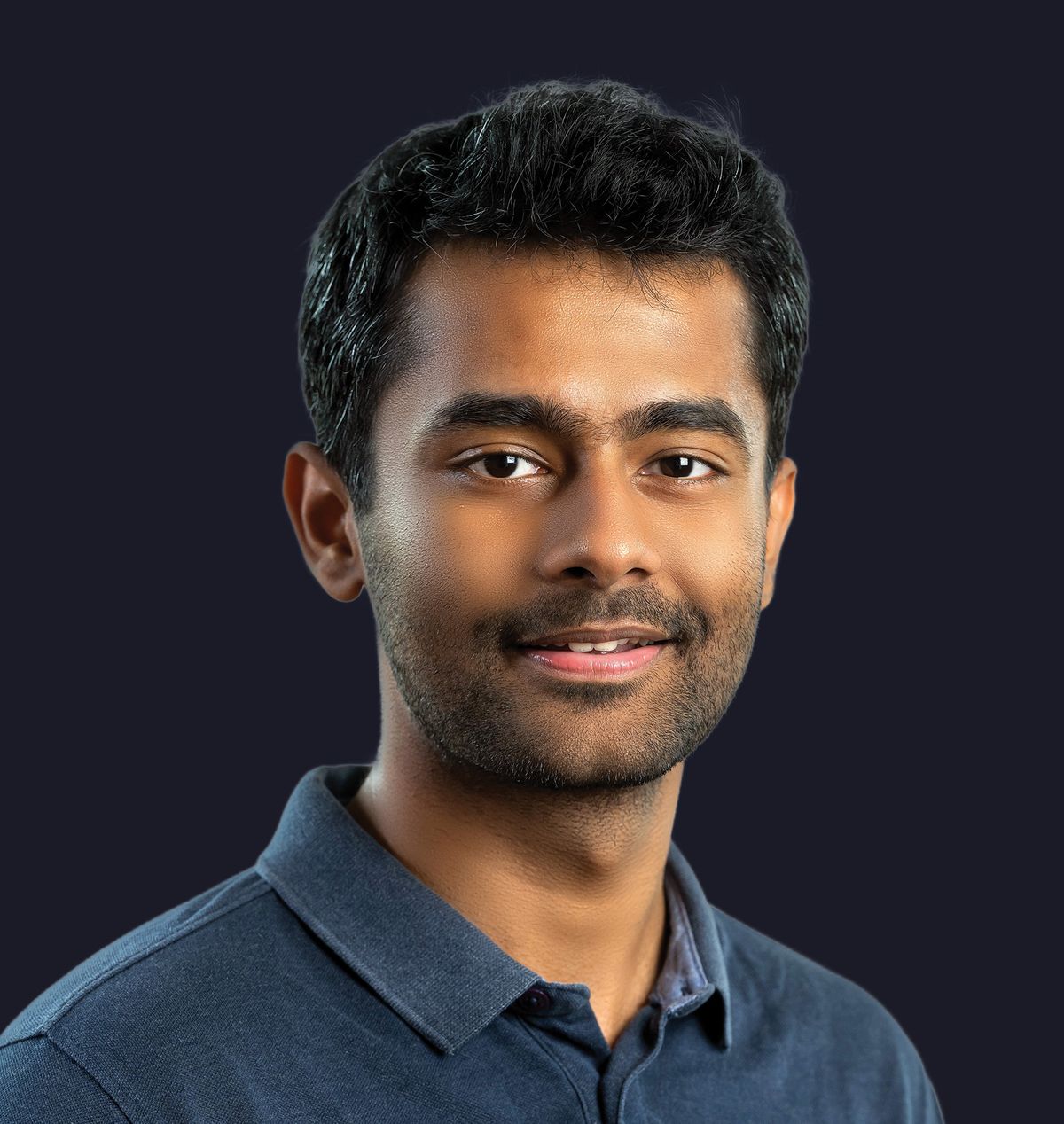
When it comes to detecting AI-generated images, Sadasivan said that things aren’t much better.8 “Training a neural network for detecting AI images is better than AI text, but still, I would say both of these are bad.” Because of the modality, it is still easier to detect when an image is fake. “Such properties which exist in nature do not exactly occur in the generated images,” said Sadasivan. AI image generators still make mistakes that provide clear evidence, even to the untrained eye, of fakery, including body parts not aligning, extra digits, or some features exhibiting more detail than neighboring elements.
AI-generated images of humans may be easy to dissect by eye now, but what about microscopy images of cells expressing fluorescently-labeled proteins that have fewer hurdles to looking realistic, even to the trained eye?
“I don't see a tool which is 100 percent reliable all the time,” said Sadasivan. “Especially in an adversarial setting—whenever the adversary has to evade, it can find a way to evade pretty much easily.” He added that the only promising AI detection system that he could imagine is one that would be interpretable, meaning it can tell the user why it thinks that the text or image is AI-generated. “If that is there, I could probably use human interference to check it and make a judgment based on humans, because most of the time these are very application specific and very sensitive.”
Kolodkin-Gal is proud of the tool that his team has developed, but also emphasized the importance of human oversight. “Proofig is not the judge,” he added. “We are highlighting potential problems.” He said it is up to the journal editors and authors to make the final decision.
- Byrne JA, Labbé C. Striking similarities between publications from China describing single gene knockdown experiments in human cancer cell lines. Scientometrics. 2017;110:1471-1493.
- Cabanac G. Chain retraction: How to stop bad science propagating through the literature. Nature. 2024;632(8027):977-979.
- Labbé C, et al. Semi-automated fact-checking of nucleotide sequence reagents in biomedical research publications: The Seek & Blastn tool. PLOS One. 2019;14(3):e0213266.
- Oste DJ, et al. Misspellings or “miscellings”—Non-verifiable and unknown cell lines in cancer research publications. 155(7):1278-1289.
- Cabanac G, Labbé C. Tortured phrases: A dubious writing style emerging in science. Evidence of critical issues affecting established journals. arXiv:2107.06751v1.
- Weidinger L, et al. Ethical and social risks of harm from Language Models. arXiv:2112.04359v1.
- Sadasivan VS, et al. Can AI-generated text be reliably detected? arXiv:2303.11156v3.
- Sadasivan VS, et al. Robustness of AI-image detectors: Fundamental limits and practical attacks. arXiv:2310:00076v2.
Membership Open House!
Enjoy OPEN access to Premium Content for a limited timeInterested in exclusive access to more premium content?