ABOVE: Using computational tools, David Baker, Demis Hassabis, and John Jumper revolutionized biology through their work on protein design and structure. Ian Haydon, UW Institute for Protein Design
In the wee hours of an October morning, David Baker, a protein biologist at the University of Washington (UW), received the most-awaited phone call in a scientist’s career. Halfway around the world, Demis Hassabis and John Jumper of Google DeepMind, an artificial intelligence (AI) company, got the same news. The three scientists had been awarded the 2024 Nobel Prize for Chemistry for their “computational work on protein design and structure.”
While AlphaFold and the subsequent AI revolution in biology garnered a lot of attention, the foundational bricks for the strides made in protein structure and design today were laid over the decades. For those fuzzy on the details, here’s a progressive timeline that led to this monumental achievement.
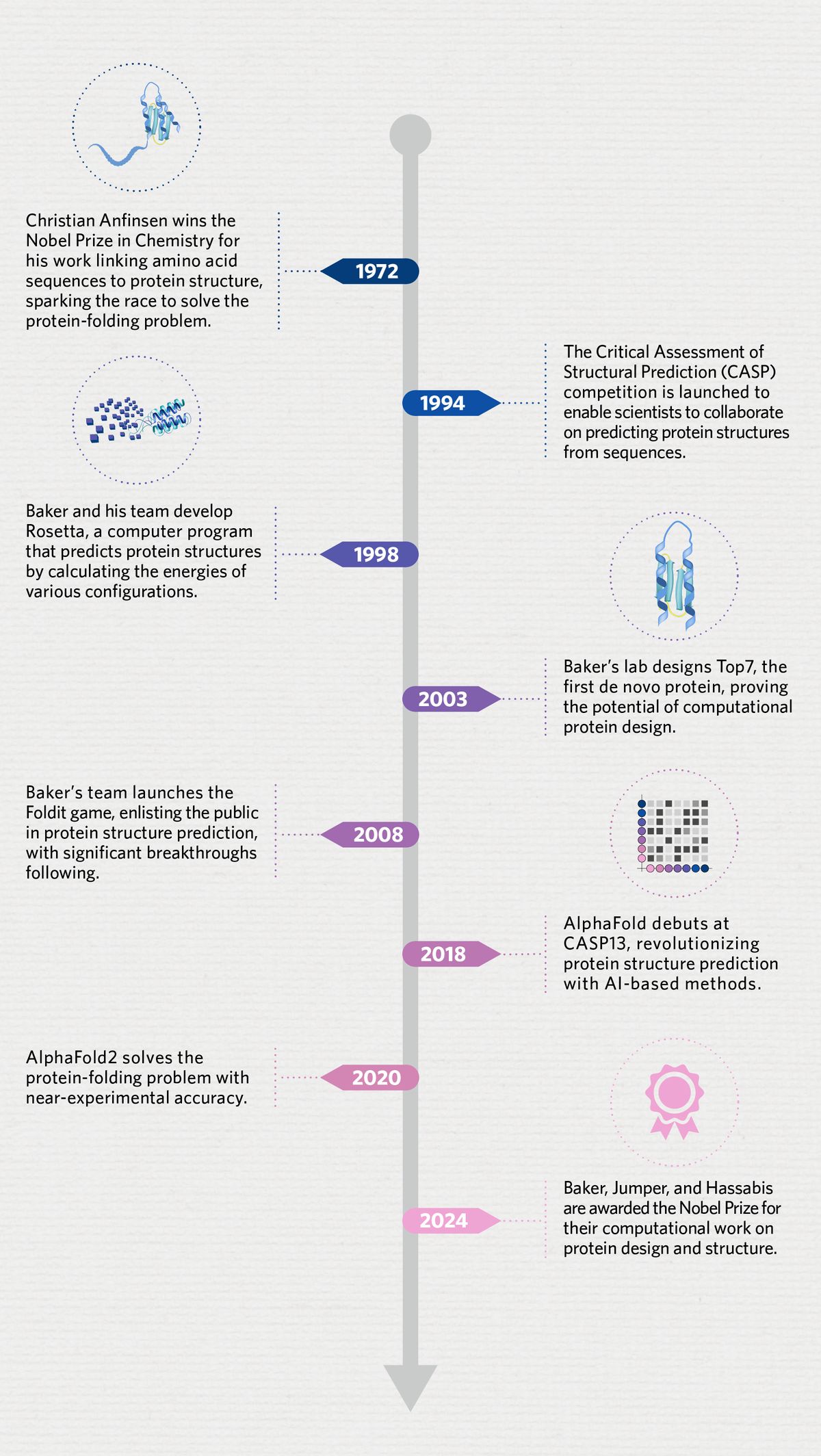
1972: Anfinsen Presents the Protein-Folding Problem
In science, it is often hard to pinpoint when a scientific problem arose. But most scientists would agree that the seed of the protein-folding problem was planted in the field of protein biology when biochemist Christian Anfinsen won the Nobel Prize in Chemistry in 1972 “for his work on ribonuclease, especially concerning the connection between the amino acid sequence and the biologically active conformation."
Anfinsen, based on his studies of the ribonuclease enzyme, proposed that all the information needed to determine the tertiary structure of a protein is encoded in its amino acid sequence. “It is certain that major advances in the understanding of cellular organization, and of the causes and control of abnormalities in such organization, will occur when we can predict, in advance, the three dimensional, phenotypic consequences of a genetic message,” said Anfinsen in his Nobel lecture.
Thus ensued the race to solve the protein-folding problem—for the next few decades, biologists attempted to reliably predict three-dimensional protein conformations from one-dimensional sequences.
1994: The CASP Competition Begins
“But going from sequence to structure has proven phenomenally difficult—biology's version of predicting the weather—at least in part because even a relatively small protein can assume a vast number of possible conformations,” explained Baker in a feature article that he wrote for The Scientist at the turn of the century.
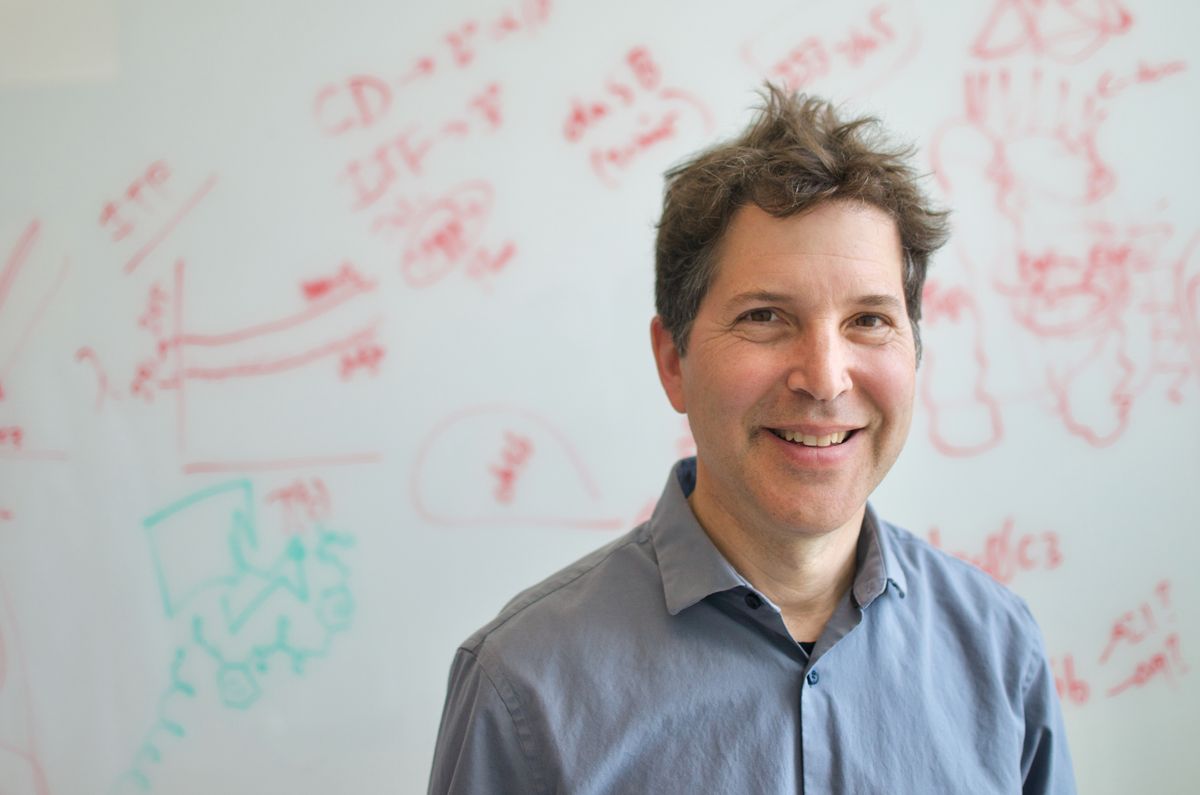
So, in 1994, University of Maryland computational biologists John Moult and Krzysztof Fidelis set up the Critical Assessment of Structural Prediction (CASP) competition to enable scientists to tackle this problem in a collaborative manner. Every couple of years, protein biologists competed to predict the structures of a few committee-selected proteins. The computational models that yielded the closest match to experimental data won.
“Proteins are made out of amino acid residues, which are made out of atoms, and you try and model all the interactions between the atoms and how they drive the protein to fold up,” Baker, who participated in the competition from its onset, explained the physical models they used back then in a previous interview.
1998: The Rosetta Program Rises
Soon, Baker and his team developed a new computer software, Rosetta, that computed the energies of different configurations to predict the optimum structure with the lowest energy.
“Eliminating unlikely structures that have, for instance, hydrophobic residues exposed to solvent, the program intelligently samples the total protein-folding landscape, testing perhaps a million or so possible conformations for the lowest energy structure,” wrote Baker in his The Scientist feature article.
The Rosetta program served a dual purpose; while it was useful for predicting protein structure, Baker also applied it for designing new proteins.
2003: Baker Reveals the First De Novo Protein
“It wasn't too long after our first successes in structure prediction that we started thinking, well, maybe instead of predicting what structure a sequence would fold up to, we could use these methods to make a completely new structure and then find out what sequence could fold to it,” said Baker in an interview earlier this year.
In 2003, Baker and his team generated the first de novo protein, a 93-amino acid protein called Top7.1 According to Baker, the fact that the X-ray structure of Top7 aligned well with their predictions demonstrated that “modern protein-design methodology can design brand-new proteins with atomic-level accuracy.”
2008: Scientists Gamify Protein Folding and Design
Baker literally made Rosetta a household name when he and his team launched Rosetta@home, an initiative that tapped into volunteers’ home computers to supplement their computing power requirements. When volunteers who offered their home computers watched the software at work, some of them provided feedback that they wished they could suggest what the program should do next.
So, Baker teamed up with computer scientists at his university, and the game Foldit was launched in 2008. Users could play the game by dragging different parts of proteins on the screen to minimize the energy—less energy meant more points. It was the perfect balance of work and play; in fact, in 2011, a group of Foldit users helped solve the structure of a protein that scientists had struggled to decode for decades.2 Citizen scientists also used the game to help design new proteins.3
2018: AlphaFold Enters the Protein Arena
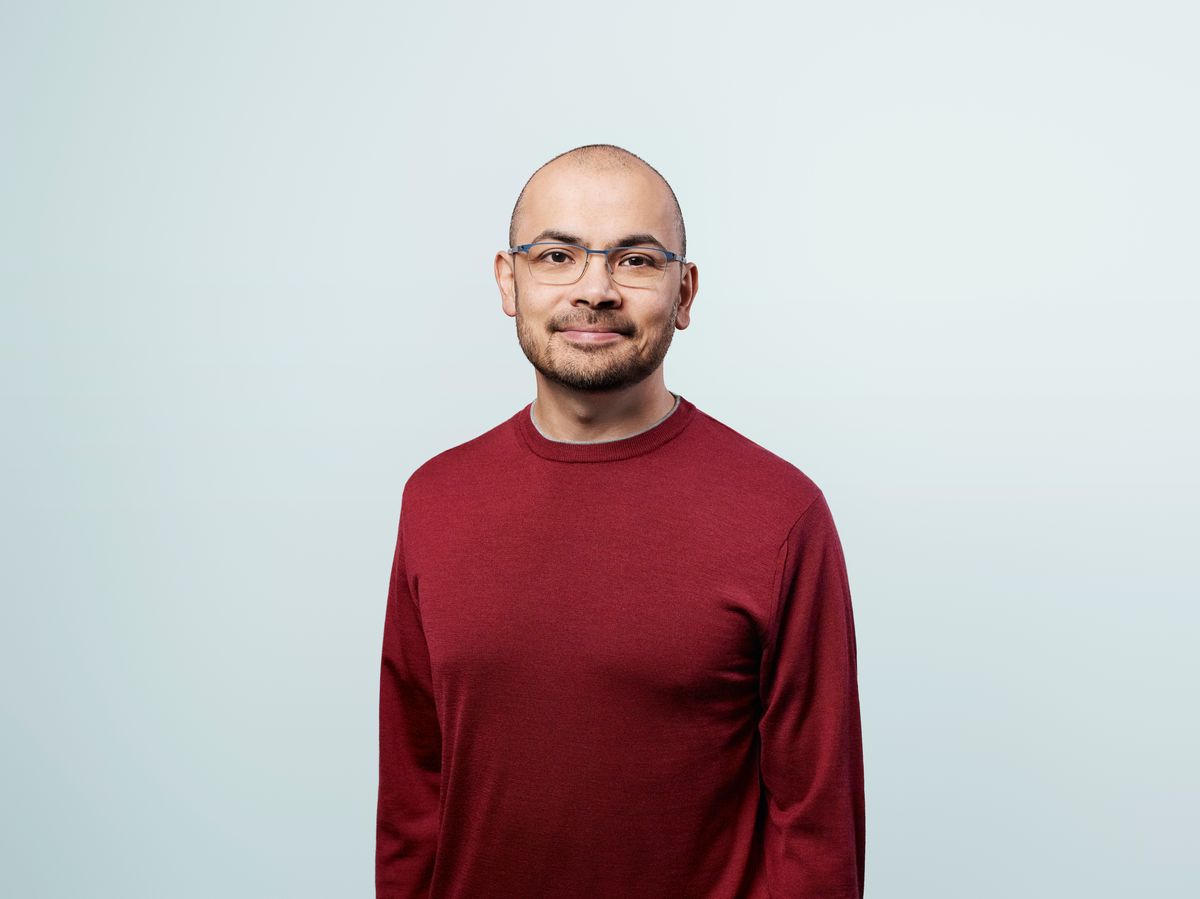
Meanwhile, Hassabis, an expert in cognitive neuroscience and cofounder of DeepMind, was also acing games. In 2016, his team applied their deep neural networks experts to launch AlphaGo, a powerful program that defeated a human champion of the board game, Go.4 Soon thereafter Hassabis turned his attention to the protein-folding problem.
The CASP competitions saw incremental progress over the years with scientists testing different computational models, but the real breakthrough came at CASP13 in 2018 when Hassabis and his team debuted their AI-based program, AlphaFold.5 Rather than modeling energy dynamics to compute structures, the machine-learning approach meant that the team trained AlphaFold using existing protein sequences and structures. After learning the rules from thousands of examples, AlphaFold could apply similar patterns to predict structures from sequences.
2020: AlphaFold2 Solves the Protein-Folding Problem
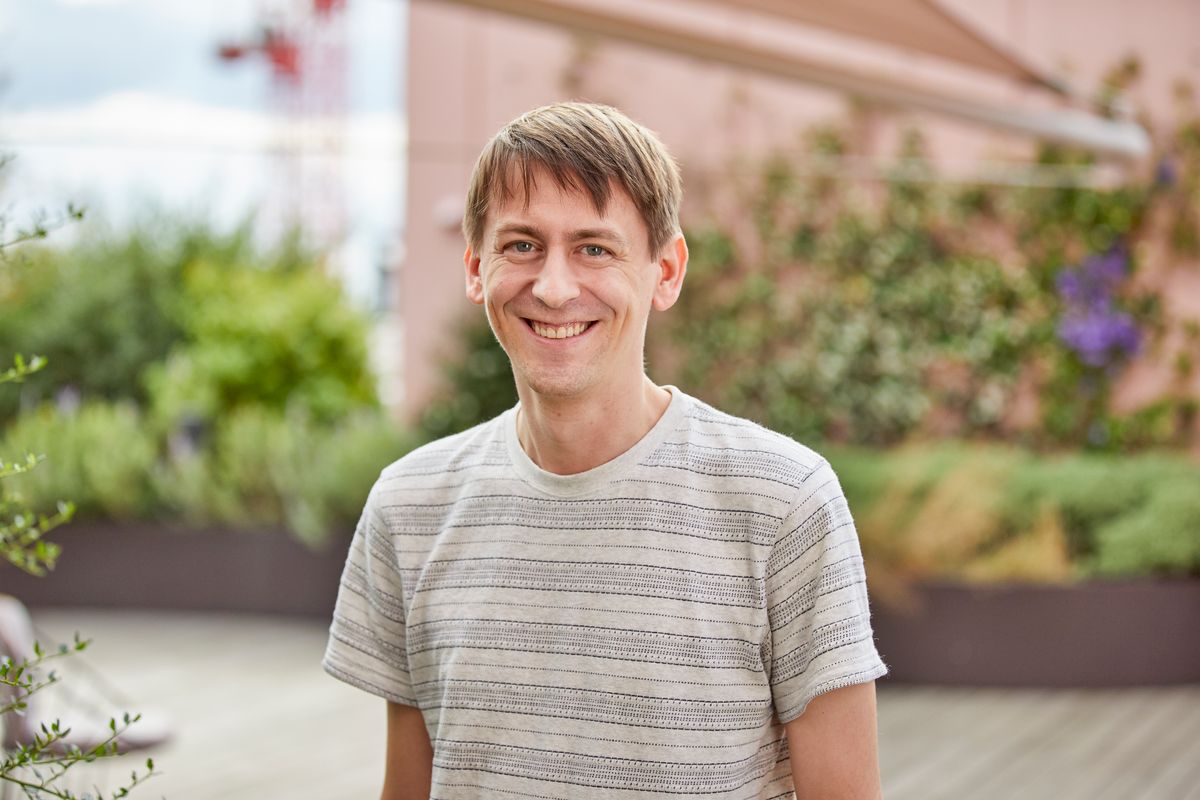
In the next CASP competition in 2020, Jumper and Hassabis came in stronger with their upleveled AlphaFold2. The new version predicted the structures of the majority of test proteins with an accuracy comparable to experimental methods.6 Such was the scale of the success of AlphaFold2, that Moult and other experts declared that the 50-year-old protein-folding problem was largely solved.
2024: Baker, Jumper, and Hassabis Bag the Nobel Prize for Chemistry
In the subsequent years, DeepMind created the AlphaFold Protein Structure Database, which now includes over 200 million structures. Access to these protein structures opened the door to a deeper understanding of their functions and potential applications across diverse areas.
“AlphaFold has already accelerated and enabled massive discoveries, including cracking the structure of the nuclear pore complex. And with this new addition of structures illuminating nearly the entire protein universe, we can expect more biological mysteries to be solved each day,” said Eric Topol, a cardiology and genomics expert at the Scripps Research Translational Institute, in a DeepMind blog article. Jumper and Hassabis won the Lasker award in 2023 for their work on AlphaFold.
As for the protein design side, after their initial success with Top7, Baker and his team developed several other de novo proteins over the years. A particularly noteworthy recent one, according to Baker, is a coronavirus vaccine (SKYCovione), developed in conjunction with Neil King at UW, which is the first de novo medicine approved for human use.7 Baker has several more projects in the pipeline, spanning diverse application areas: targeted therapeutics, plastic-degrading enzymes, and carbon dioxide-fixing proteins.
“The proteins in nature evolved under the constraints of natural selection. So, they solve all the problems that were relevant for natural selection during evolution. But now, we can make proteins specifically for 21st-century problems. That is what is really exciting about the field,” said Baker.
- Kuhlman B, et al. Design of a novel globular protein fold with atomic-level accuracy. Science. 2003;302(5649):1364-1368.
- Khatib F, et al. Crystal structure of a monomeric retroviral protease solved by protein folding game players.Nat Struct Mol Biol. 2011;18(10):1175-1177.
- Koepnick B, et al.De novo protein design by citizen scientists. Nature. 2019;570(7761): 390-394.
- Silver D, et al.Mastering the game of Go with deep neural networks and tree search.Nature. 2016;529(7587):484-489.
- Senior AW, et al.Improved protein structure prediction using potentials from deep learning. Nature. 2020;577(7792):706-710.
- Jumper J, et al. Highly accurate protein structure prediction with AlphaFold. Nature. 2021;596(7873):583-589.
- Walls AC, et al. Elicitation of potent neutralizing antibody responses by designed protein nanoparticle vaccines for SARS-CoV-2. Cell. 2020;183(5):1367-1382.e17.